

Disaster Management
Estimates of exposure to the 100-year floods in CONUS



The objective of this study is to conduct a comparative assessment of exposed population within 100-year floodplains in the CONUS. To better capture the heterogeneity of population distribution at micro-level, national building footprints, trimmed by National Land Use Dataset 2010 (NLUD 2010) and OpenStreetMap Land Use polygons, are used to disaggregate block group population from the latest ACS 5-year estimates (2013-2017). The findings from this national assessment provide valuable benchmark information regarding current flood exposure (100-year flood) in the CONUS by answering the following questions: 1) how many people are exposed? 2) who are they? 3) how are they distributed? 4) how do the estimations from other floodplains differ from FEMA floodplain?
Understand hurricane-prone human settlement using nighttime RS

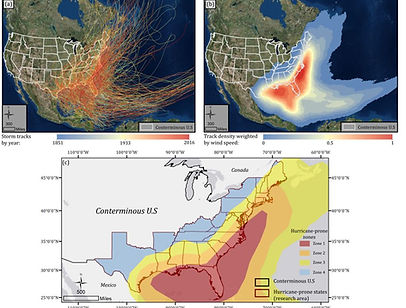
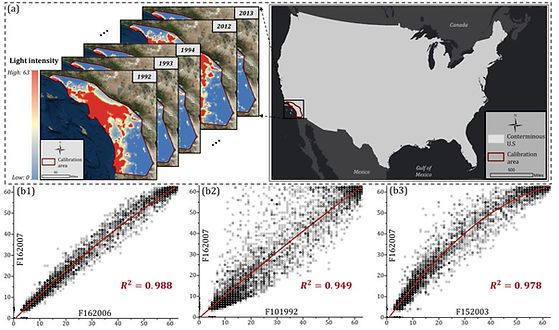
This study uses the DMSP/OLS nighttime light (NTL) to shed lights on human settlement
development in areas with different levels of hurricane proneness from 1992 - 2013. The DMSP OLS NTL data from six satellites are intercalibrated and desaturated with AVHRR and MODIS optical imagery to derive the vegetation-adjusted NTL urban index (VANUI). The results clearly exhibit a north-south and inland-coastal discrepancy of human settlement.
It also reveals that both the zonal extent and zonal increase rate of human settlement positively correlate with hurricane proneness levels during the investigated period. (Huang et al., In prep.)
Reconstructing flood inundation by integrating RS and SS
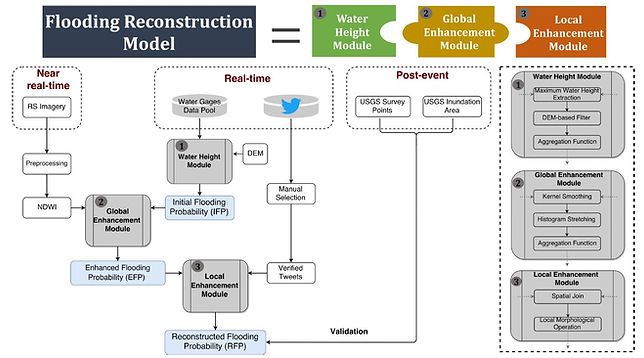
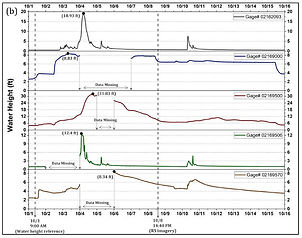
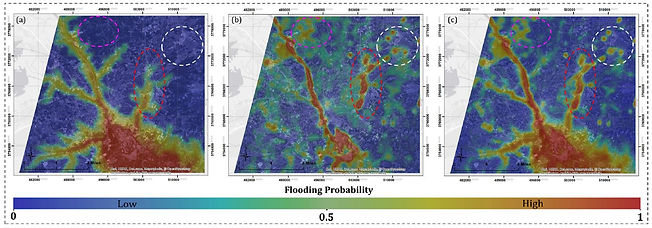
This paper proposed a flood inundation reconstruction model by enhancing the NRT normalized difference water index derived from remote sensing imagery with the RT data including stream gauge readings and social media. Results suggest that by enhancing NRT, the proposed flood inundation probability model renders a more robust, spatially enhanced flood probability index for emergency responders to quickly identify areas in need of urgent attention (Huang et al., 2018)
Near real-time flood mapping

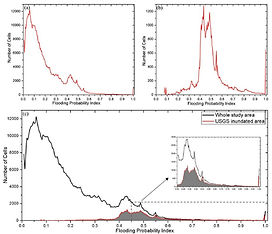

This study proposed a novel approach to retrieve near real-time flood probability map by integrating the post-event remote sensing data with the real-time (VGI). Results indicate that, by adding the wetness information from post-event satellite observations, the proposed model could provide near real-time flood probability distribution with real-time social media. (Huang et al., 2018)
Automated tagging of flood-related tweets

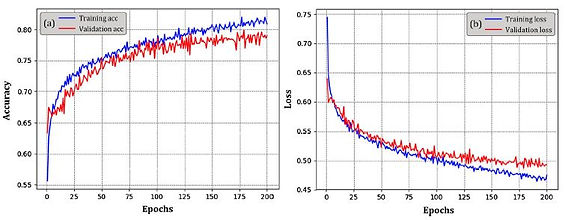
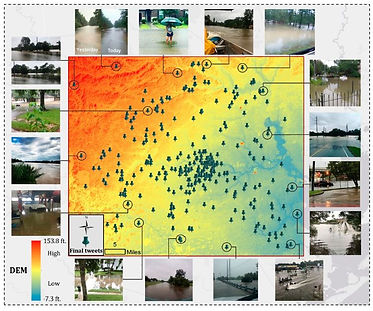
This study presented an automated flood tweets extraction approach by mining both visual and textual information a tweet contains. The results indicated that coupling CNN classification results with flood sensitive words allows a significant increase in precision while keeps the recall rate in high level. The method proposed contributes to higher spatio-tempoeal relevance to the flood. (Huang et al., 2018)
Visual-textual deep learning framework



Two convolutional neural networks (CNNs), the Inception-V3 CNN and word embedded CNN, are applied to extract visual and textual features, respectively, from social media posts. Well-trained on our designed training sets, the extracted visual and textual features are further concatenated to form a fused feature to feed the final classification process. The results suggest that both CNNs perform remarkably well in learning visual and textual features. The fused feature proves that additional visual feature leads to more robustness compared with the situation where only textual feature is used. (Huang et al., In review)